Artificial intelligence and machine learning in hemostasis and thrombosis
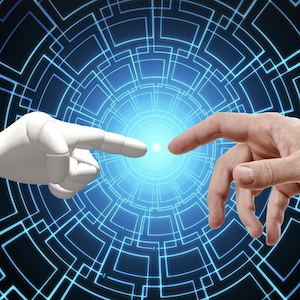
Published: December 30 2023
Abstract Views: 2249
PDF: 451
Publisher's note
All claims expressed in this article are solely those of the authors and do not necessarily represent those of their affiliated organizations, or those of the publisher, the editors and the reviewers. Any product that may be evaluated in this article or claim that may be made by its manufacturer is not guaranteed or endorsed by the publisher.
All claims expressed in this article are solely those of the authors and do not necessarily represent those of their affiliated organizations, or those of the publisher, the editors and the reviewers. Any product that may be evaluated in this article or claim that may be made by its manufacturer is not guaranteed or endorsed by the publisher.
Similar Articles
- Giovanni de Gaetano, Artificial intelligence, platelets and aspirin , Bleeding, Thrombosis and Vascular Biology: Vol. 3 No. 1 (2024)
- Paolo Simioni, Vittorio Pengo, Paolo Prandoni, Thrombosis and hemostasis at the University of Padua: a reappraisal on the occasion of its 800th year of history , Bleeding, Thrombosis and Vascular Biology: Vol. 1 No. 3 (2022)
- Rushad Patell, Jeffrey I. Zwicker, Rohan Singh, Simon Mantha, Machine learning in cancer-associated thrombosis: hype or hope in untangling the clot , Bleeding, Thrombosis and Vascular Biology: Vol. 3 No. s1 (2024): 12th ICTHIC
- Luca Barcella, Chiara Ambaglio, Paolo Gritti, Francesca Schieppati, Varusca Brusegan, Eleonora Sanga, Marina Marchetti, Luca Lorini, Anna Falanga, Long-term persistence of high anti-PF4 antibodies titer in a challenging case of AZD1222 vaccine-induced thrombotic thrombocytopenia , Bleeding, Thrombosis and Vascular Biology: Vol. 2 No. 2 (2023)
- Rita Carlotta Santoro, Roberto Minici, Marzia Leotta, Mariapia Falbo, Lucia Concetta Elia, Francesca Leo, Antonella Ierardi, Alessandra Strangio, Simona Prejanò, Vaccine-induced immune thrombotic thrombocytopenia following ChAdOx1 nCoV-19 vaccine: report of two cases , Bleeding, Thrombosis and Vascular Biology: Vol. 1 No. 2 (2022)
- Juan Eirís, Marina Suárez-Terrón, Pablo Granados, David Martínez-Campuzano, Ana Rosa Cid, Saturnino Haya, Santiago Bonanad, Allopurinol-induced acquired von Willebrand syndrome , Bleeding, Thrombosis and Vascular Biology: Vol. 2 No. 4 (2023)
- Daniela Poli, Walter Ageno, Emilia Antonucci, Salvatore Bradamante, Eugenio Bucherini, Paolo Chiarugi, Antonio Chistolini, Benilde Cosmi, Anna Falanga, Antonio Insana, Domenico Lione, Rosa Maria Lombardi, Giuseppe Malcangi, Rossella Marcucci, Giuliana Martini, Lucilla Masciocco, Carmelo Paparo, Daniele Pastori, Simona Pedrini, Vittorio Pengo, Pasquale Pignatelli, Andrea Toma, Sophie Testa, Gualtiero Palareti, Management of anticoagulation in atrial fibrillation patients in Italy: insight from the Atrial Fibrillation-Survey on Anticoagulated Patients Register (AF-START) , Bleeding, Thrombosis and Vascular Biology: Vol. 2 No. 2 (2023)
- Roberto Mario Santi, Annamaria Fulghesu, Ezio Zanon, Erica De Candia, Elvira Grandone, Giancarlo Di Renzo, Claudia Succu, Valentina Tosto, Vincenzina Bruni, Paolo Gresele, Diagnosis and management of abnormal uterine bleeding in adolescence , Bleeding, Thrombosis and Vascular Biology: Vol. 3 No. 1 (2024)
- Francesco Marongiu, Elvira Grandone, Silvia Marongiu, Antonella Mameli, Doris Barcellona, Stroke in women: anticoagulation in a complicated puzzle , Bleeding, Thrombosis and Vascular Biology: Vol. 3 No. 2 (2024)
- Bianca Clerici, Mariangela Scavone, Gian Marco Podda, Recent advances in classic heparin-induced thrombocytopenia (HIT), autoimmune HIT, spontaneous HIT, and vaccine-induced immune thrombotic thrombocytopenia , Bleeding, Thrombosis and Vascular Biology: Vol. 3 No. 2 (2024)
1-10 of 78
Next
You may also start an advanced similarity search for this article.